Generative AI Vs. Traditional AI: Which is Better
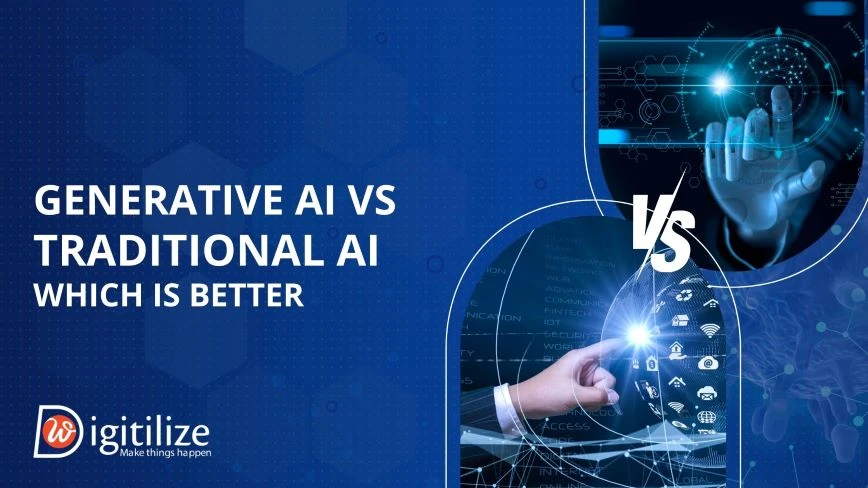
Traditional AI
Traditional AI, sometimes called expert systems or rule-based AI Artificial intelligence (AI) is a subclass of AI that executes tasks and makes decisions using preset rules and algorithms. As opposed to traditional AI, which emphasizes one activity, general AI aims to demonstrate human-like intelligence across multiple activities. As a result, it works well in fields where the rules are clear and constant, such industrial automation, expert medical diagnosis, and AI for gaming. To put it simply, traditional AI aids in problem-solving, task automation, and prediction.Benefits of Traditional AI
-
Specified and comprehensible outcomes
-
Effectiveness in completing particular tasks
-
A proven track record in fields like automation and robotics
-
Ideal for assignments with a large amount of labelled data
Traditional AI’s drawbacks
-
Restricted Flexibility:
-
Scalability
-
Absence of Generalisation:
Traditional AI is rapidly growing, much like generative AI.
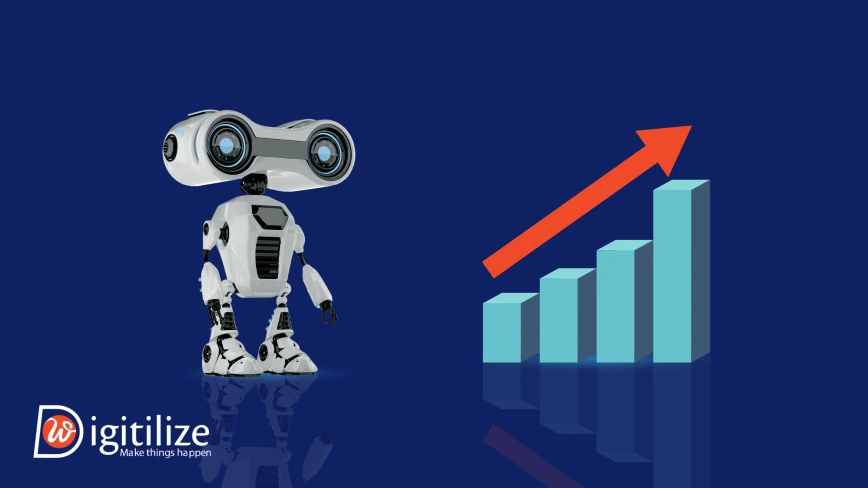
-
Generative Intelligence
Benefits of Generative AI
-
Increased imagination and original content creation
-
Innovative uses across several sectors
-
Possibility of producing innovative art and media
Limitations of Generative AI
- Complexity: Generative AI models may be computationally demanding to train and fine-tune, requiring substantial quantities of data and processing power.
- Lack of openness: It might be difficult to understand how deep learning models operate internally, which raises questions about accountability and openness.
The Generative AI Future Prospects
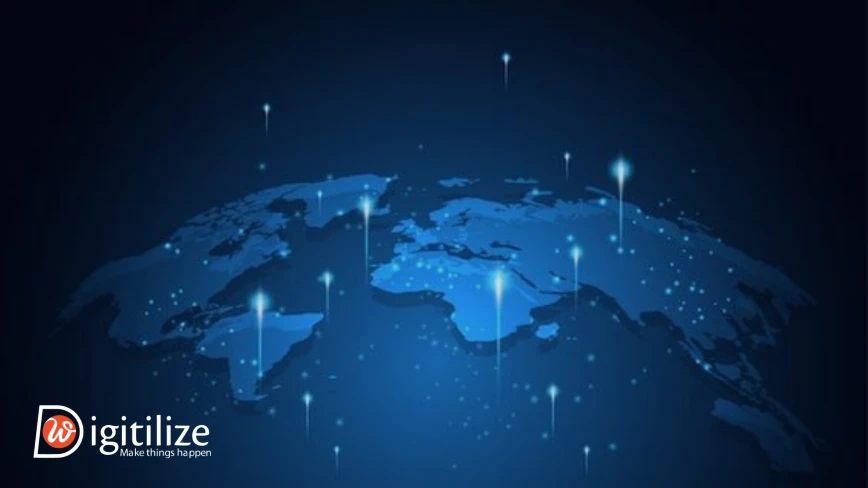
- PaLM from Google AI
- LaMDA from Meta AI
- GPT-3 from OpenAI
- Gopher from DeepMind
- MS Research’s Megatron-Turing Natural Language
- Google AI is using Pam to build new code and song generators, among other creative tools.
- Meta AI is using LaMDA to develop new social capabilities, such the ability to have chatbot conversations that seem more realistic and interesting.
- DeepMind is using Gopher to develop new medical tools, such a system that might help doctors diagnose patients more accurately.
- Microsoft Research is using Megatron-Turing NLG to develop new manufacturing tools, such a system that helps designers create new things more efficiently.
AI Comparison between Traditional AI and Generative AI
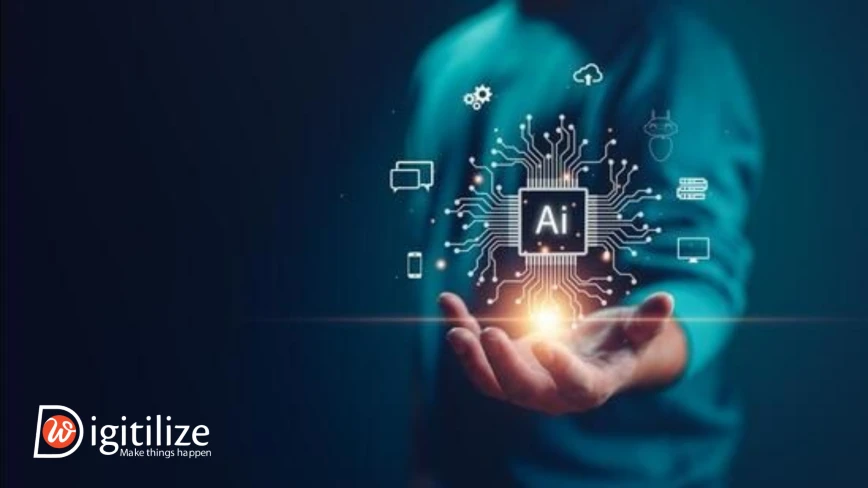
Rule-based vs. data-driven methods:
Traditional AI: To enable a system to carry out a particular function, conventional AI relies on rules and explicit instructions. Human specialists have created these guidelines based on their knowledge of the relevant issue domain. In order to make judgements and produce results, traditional AI systems adhere to certain guidelines. Generative AI: This type of AI bases its AI decision-making processes on data. It uses machine learning methods, like as deep neural network architectures, to extract patterns and structures from massive information. By identifying underlying patterns and correlations in the data, generative AI models learn from the data and produce new content without the need for explicit rules.Unsupervised vs. Supervised Education
Traditional AI: Supervised vs Unsupervised Learning In supervised learning, which is a common technique used in traditional AI. Also the AI model selection is trained using labelled data that contains inputs and the matching outputs. Based on these labelled instances, the model learns how to translate inputs to certain outputs. So, for it to learn and produce reliable predictions, human annotations are necessary. Generative AI: This type of artificial intelligence methods is capable of both supervised and unsupervised learning, however it performs best in situations involving unsupervised learning. Unsupervised learning involves training the model on unlabeled data so that it may discover underlying structures and patterns on its own without direct human assistance. Generative AI is effective in unsupervised environments because of its capacity to produce new information and media.Generative vs. discriminative models
Traditional AI Discriminative models are usually used in traditional AI. Discriminative models are trained to discern between several data classes or categories. In image classification, for instance, a discriminative model learns to categorise photos into particular groups (such dogs or cats) according to their properties. Generative models are used in generative AI. In addition to producing new samples that closely resemble the original data. The generative models can understand the underlying probability distribution of the data. One well-known generative model that may produce realistic visuals that mimic real-world instances is called a Generative Adversarial Network (GAN).Flexibility and inventiveness in Generative AI
Traditional AI since the former is programmed to do certain tasks and is not as flexible or creative as the latter. So, it adheres to preset guidelines and cannot create original material or adjust to novel circumstances without the direct involvement of a person. Generative AI: Because it can produce original material, generative AI evaluation criteria creativity and flexibility. It may produce original and imaginative works of art, including new writings, pictures, music, and more. Furthermore, AI technology evaluation may produce material that conforms to fresh patterns or modifications in the input data and adjusts to various data distributions.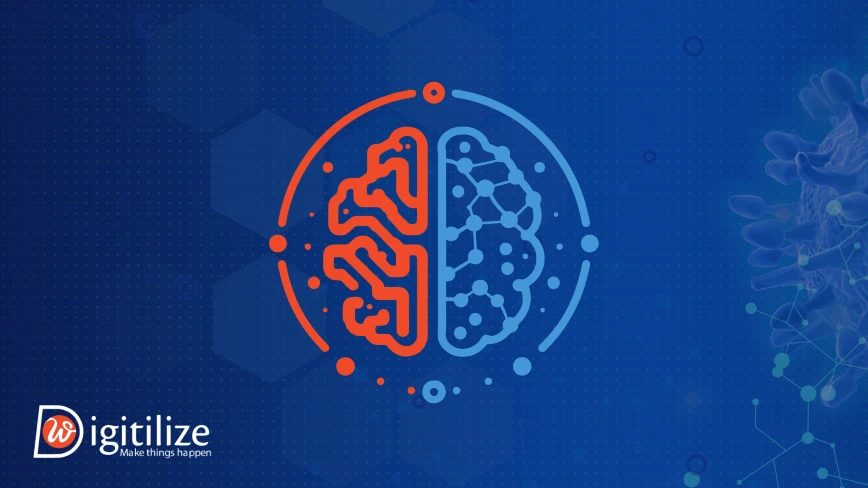